In AgTech, big data means collecting everything. The temperature of the air as you plant, the moisture of the kernel as you harvest, the exact coordinate of each weed you find; it all gets absorbed into the digital cloud, analyzed, and processed. The end result is that we know what seeds grow the best in different conditions, statistically speaking.
Often this leads to homogenized recommendations. Sure, the corn hybrid ‘SKY-3122’ may rate the best in some parts of the country, but does that mean it’s best for your customers’ farms? The all-time favorite food across the US may be a good old cheeseburger fresh off the grill, but try to make everyone eat one and you’ll end up with a lot of unhappy vegetarians.
The end goal of all this data aggregation
Customized recommendations are the end goal of aggregated data. Given a weather prediction and the soil types for a field, we can predict the seed that’ll give you the highest yield. With enough data, we can divide the field into zones and predict how much of that seed you should plant in each square foot. As planter technology advances, we’re starting to sow entirely different seeds in different parts of the field to take advantage of the unique properties of the land. With drones taking flight, we can target individual plants for pesticide applications.
Seeing the field for the corn stalks
There’s no question that the volume of data and customized applications have advanced sustainability and food production dramatically. Yet, as our ability to refine these recommendations has grown, so has another problem: information overload that’s made it hard to see the field for the stalks.
Anyone who has tended a farm knows the logistical nightmares behind getting everything planted in that sudden timeframe in spring, or trying to find the sweet spot in the fall for harvesting your crop. Now multiply that tenfold as suddenly you need to account for which fields need to be harvested first because of the performance of a particular seed that year, or having to load up three times as many bags of seeds because now each field has three different varieties. Data has become AgTech’s greatest asset and its Achilles heel.
Are automated recommendations the best choice?
In one sense, yes. We need automated recommendations that can take all this data to make smarter recommendations that take the bigger picture into account. Having worked on dispatch software for equipment managers, communication software, ordering software, portfolio recommendations, and generally the entire pipeline of precision agriculture, we’ve seen a need to connect each of these components to provide a higher-order, holistic approach.
If we’re going to suggest that farmers plant a half-dozen different varieties across a dozen fields, we need to have a sense of how that inventory will be transported, and optimize the field-by-field to account for travel times. If we want to provide variable-rate prescriptions to take advantage of the soil, we need to know exactly what was purchased, what was actually planted, and what was actually harvested to prove at the end of the year that all the effort was worth it.
What data can’t tell you
Which would you trust when a plane’s engine goes out: the software that stabilizes speed, height and direction? The autopilot’s hydraulics, mechanical and electrical systems? I’d rather trust the pilot and crew to take control of the plane, based on their years of training, experience – and gut instinct.
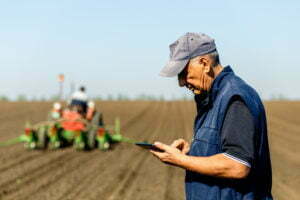
Farmers have a world of data in their hands, but often too much
With all the automation in AgTech, we’re in danger of losing the spark of human instinct and experience. There will always be quirks and exceptional circumstances that should override our reliance on science. An AI-driven recommendation system may suggest a drought-tolerant hybrid for a field, but a grower may know that doesn’t make sense, despite what the historical data may say. Or perhaps there’s a particular invasive species that is on the move, one that the system could have no way of predicting. The decision-making capability of a grower cannot be replaced by any amount of data and automated recommendations. After all, human instincts have evolved over centuries of learning and survival.
Skyward has been working on allowing greater control and insight of all the algorithms and models that drive the recommendations. To meld the algorithm and the human instinct, we look at a few things:
- Rationale — why the algorithm chose a particular recommendation
- Precision insight — how does this recommendation affect the specific area to which it is applied
- Portfolio overview — how does the individual recommendation incorporate into your priorities across the whole farm
- Prediction — with different scenarios, what are the expected outcomes
With these elements in hand, you can trust that the process is focused on specific opportunities that fit into broader priorities.
When are new data-driven applications most successful?
Data-based innovations succeed when they take some of the guesswork out of a farmer’s decision, create more efficiencies , reduce expenses and waste and aggregate data for a single source of truth.
Collecting, extracting, consolidating and visualizing data has enabled our agribusiness clients to deliver solutions to farmers that have done just that. The algorithms and data we have generated have led to:
- Localized weather predictions, both short-term and climatological
- Trial runs for proof of performance
- Soil sampling and management zone creation
- Precision Scouting
- Satellite imagery interpretation
Want to know how data has been used successfully and when and why it’s failed? We’d be happy to walk you through some common use cases. There’s no cost to start thinking about what’s possible.